The Umbra Database System
Umbra is an efficient relational database management system that is incredibly fast for all use cases.
Umbra is designed to process data at line-speed under all circumstances. In memory, we process hundreds of gigabytes per second while gracefully falling back to flash-based storage. Using data-centric code generation, parallel execution, and advanced query optimization techniques, Umbra executes the most complex queries with ease.
Try Web Interface Download Umbra PublicationsFeatures
Disk-Based with In-Memory Performance
Umbra uses a low-overhead buffer manager based on LeanStore to support larger-than-memory workloads. This provides unparalleled performance for workloads on data that is cached in memory, while degrading gracefully when the data does not fit in RAM.
Data-Centric Code Generation
Umbra generates optimized code, even for complex expressions in a custom intermediate representation that is optimized for low-latency query execution. Direct machine code generation starts query execution instantly, while long-running queries are automatically accelerated using the LLVM optimizing compiler.
Fully Parallel Query Execution
Our query execution is designed to scale to hundreds of cores and take full advantage of parallel compute resources. In our morsel-driven parallelism, we use contention-free parallel algorithms that provide near-perfect scaling for large analytic queries and still low latency for short transactional queries.
No Compromises
Umbra ensures transactional ACID properties through in-memory optimized multi-version concurrency control. We also use full-precision fixed-point arithmetic and unconditional overflow checking for always-correct results.
Computational Database beyond SQL
Umbra provides an efficient approach to user-defined functions. The system automatically parallelizes user functions, creates deep integration into generated code, and ensures morsel-driven execution. This makes it easy to extend Umbra with advanced operations, e.g., gradient-descent and k-means algorithms.
Versatile Join Implementations
Umbra's data structures are designed for parallel processing that scales to hundreds of cores. Groupjoins enable efficient computation of aggregates, worst-case optimal joins handle complex queries on graph structured data, and range joins efficiently evaluate queries with conditions on location or time intervals.
Advanced Statistics
To precisely estimate result sizes and query costs, Umbra combines sketches and sampling. Reservoir sampling allows always up-to-date statistics even during live inserts. In addition, numerical statistics can even estimate derived values of aggregates.
Complex SQL Queries
Umbra supports the efficient execution of arbitrarily complex SQL queries in the PostgreSQL dialect. With our advanced optimization techniques, we achieve excellent performance for complex correlated subqueries, advanced window functions, and complex nested types such as JSON data.
Team
From the Creators of HyPer
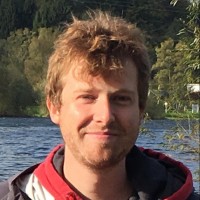
Maximilian Bandle
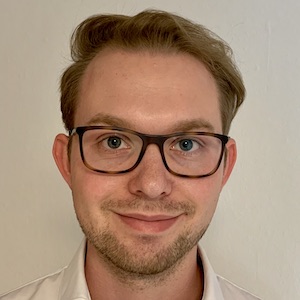
Alexander Beischl
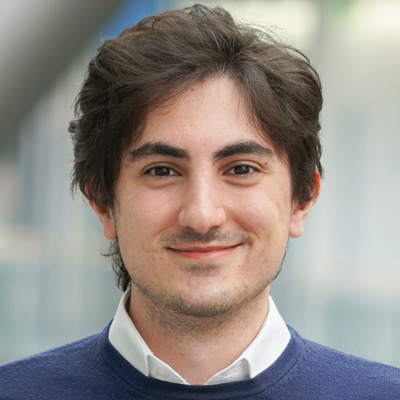
Altan Birler
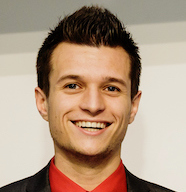
Dominik Durner
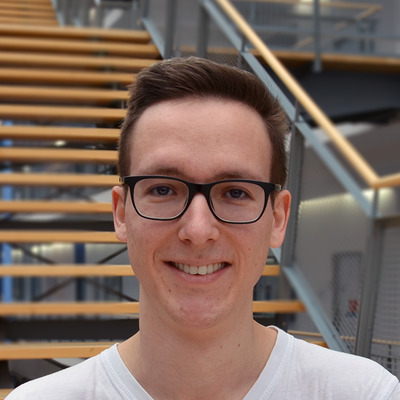
Simon Ellmann
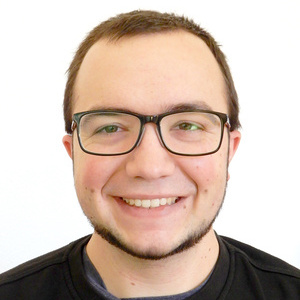
Philipp Fent
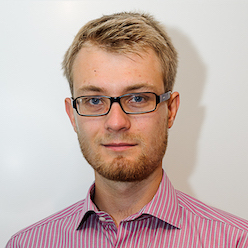
Michael Freitag
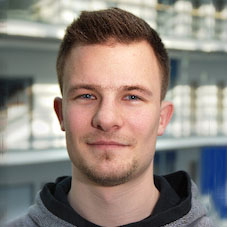
Timo Kersten
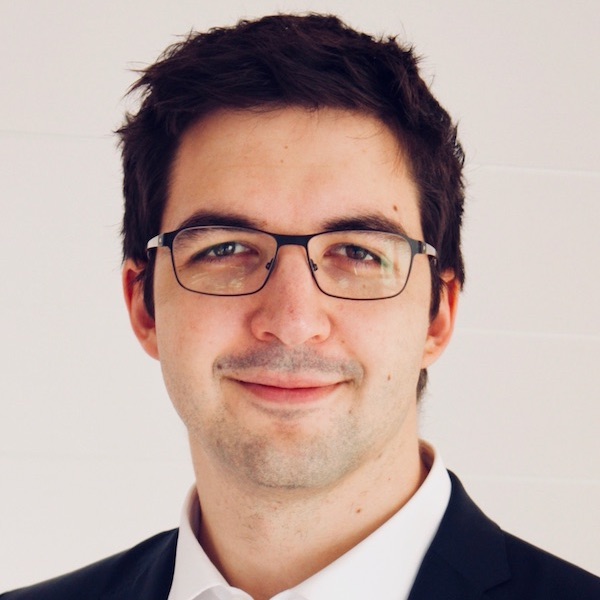
André Kohn
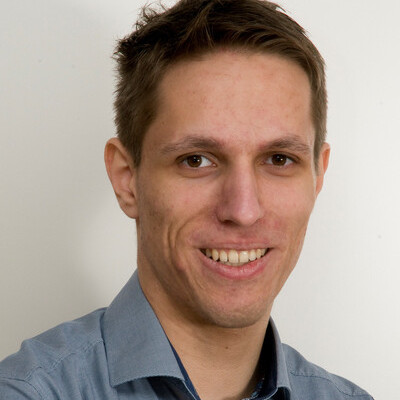
Stefan Lehner
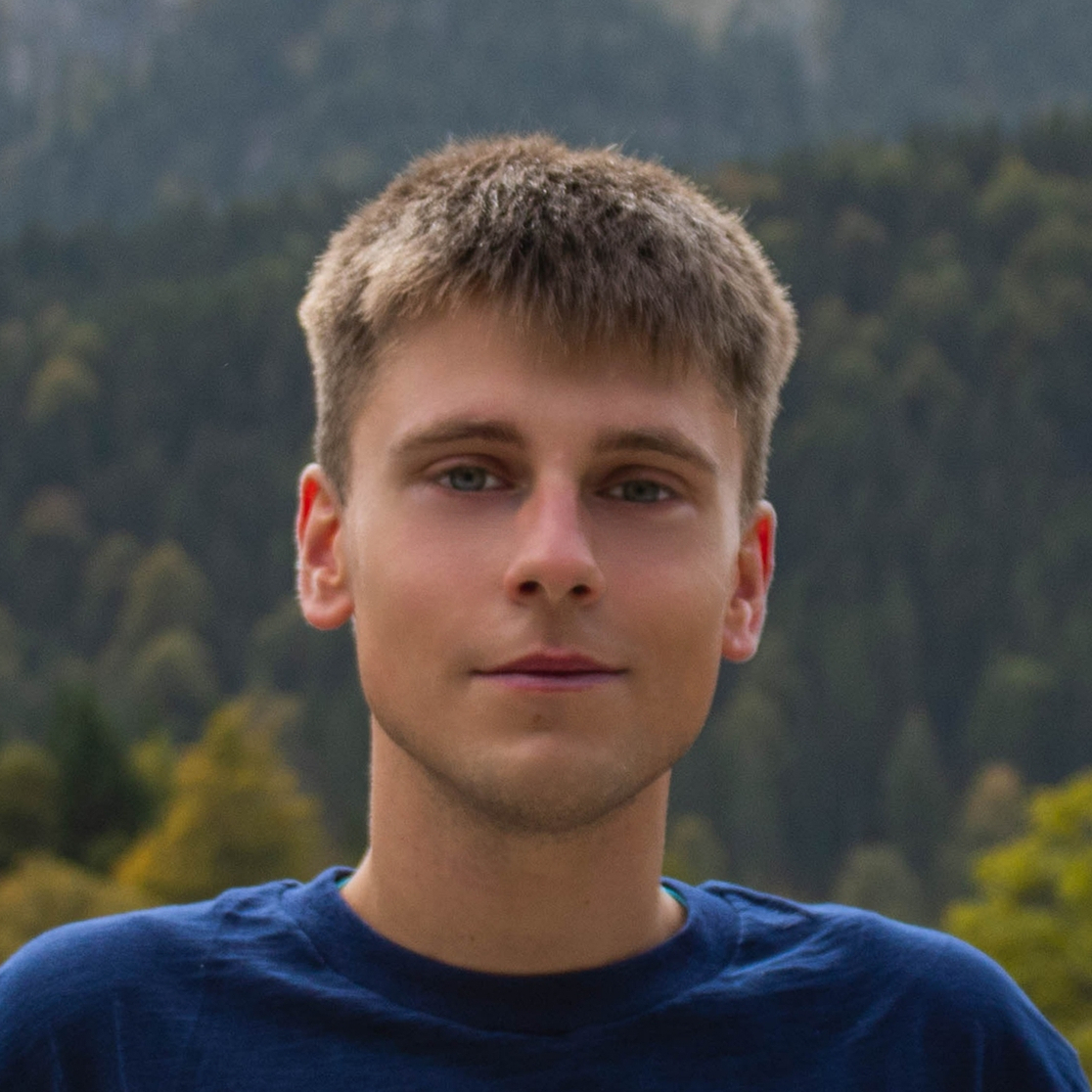
Mykola Morozov
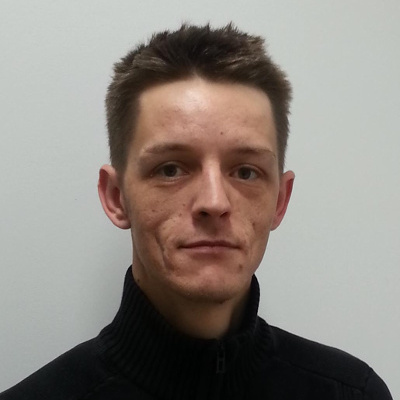
Bernhard Radke
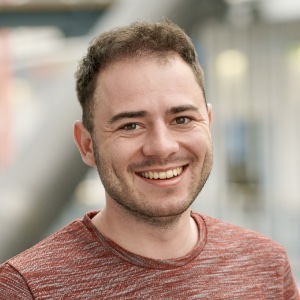
Maximilian Reif
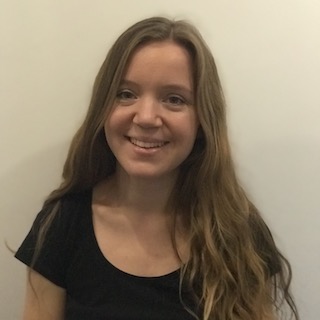
Alice Rey
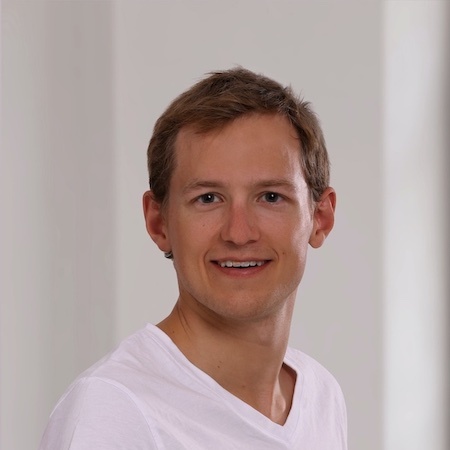
Adrian Riedl
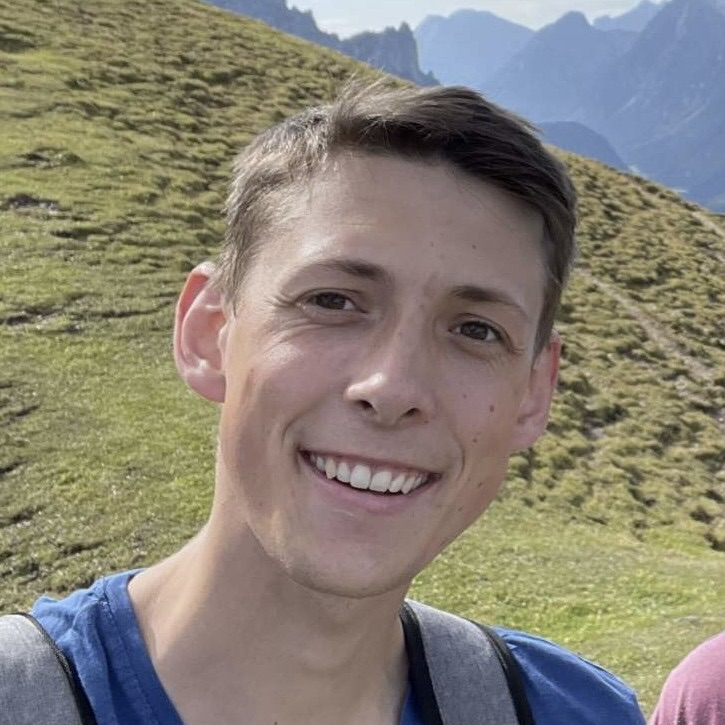
Maximilian Rieger
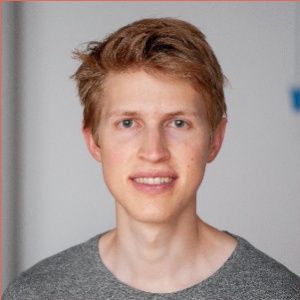
Felix Rinderer
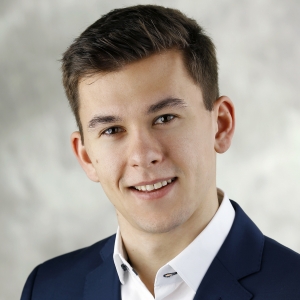
Tobias Schmidt
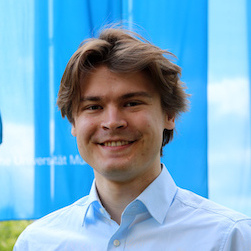
Maximilian Schüle
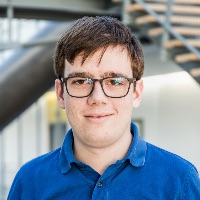
Moritz Sichert
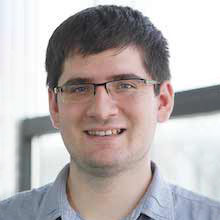
Lukas Vogel
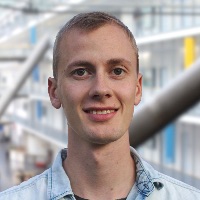
Christian Winter
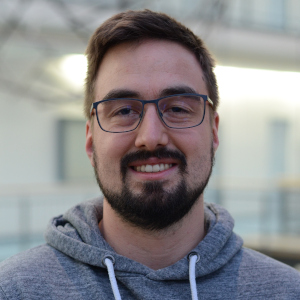
Michael Zinsmeister
Publications
Understand Umbra
Foundational publications
2020
Umbra: A Disk-Based System with In-Memory Performance
CIDR 2020 | Thomas Neumann and Michael Freitag | January 13, 2020
Abstract
The increases in main-memory sizes over the last decade have made pure in-memory database systems feasible, and in-memory systems offer unprecedented performance. However, DRAM is still relatively expensive, and the growth of main-memory sizes has slowed down. In contrast, the prices for SSDs have fallen substantially in the last years, and their read bandwidth has increased to gigabytes per second. This makes it attractive to combine a large in-memory buffer with fast SSDs as storage devices, combining the excellent performance for the in-memory working set with the scalability of a disk-based system. In this paper we present the Umbra system, an evolution of the pure in-memory HyPer system towards a disk-based, or rather SSD-based, system. We show that by introducing a novel low- overhead buffer manager with variable-size pages we can achieve comparable performance to an in-memory database system for the cached working set, while handling accesses to uncached data gracefully. We discuss the changes and techniques that were nec- essary to handle the out-of-memory case gracefully and with low overhead, offering insights into the design of a memory optimized disk-based system.
All publications
2024
Robust Join Processing with Diamond Hardened Joins
VLDB 2024 | Altan Birler, Alfons Kemper, and Thomas Neumann | August 26, 2024
Abstract
Join ordering and join processing has a huge impact on query execution and can easily affect the query response time by orders of magnitude. In particular, when joins are potentially growing n:m joins, execution can be very expensive. This can be seen by examining the sizes of intermediate results: If a join query produces many redundant tuples that are later eliminated, the query is likely expensive, which is not justified by the query result. This gives the query a diamond shape, with intermediate results larger than the inputs and the output. This occurs frequently in various workloads, particularly, in graph workloads, and also in benchmarks like JOB. We call this issue the diamond problem, and to address it, we propose the diamond hardened join framework, which splits join operators into two suboperators: Lookup & Expand. By allowing these suboperators to be freely reordered by the query optimizer, we improve the runtime of queries that exhibit the diamond problem without sacrificing performance for the rest of the queries. Past theoretical work such as worst-case optimal joins similarly try to avoid huge intermediate results. However, these approaches have significant overheads that impact all queries. We demonstrate that our approach leads to excellent performance both in queries that exhibit the diamond problem and in regular queries that can be handled by traditional binary joins. This allows for a unified approach, offering excellent performance across the board. Compared to traditional joins, queries’ performance is improved by up to 500x in the CE benchmark and remains excellent in TPC-H and JOB.
Two Birds With One Stone: Designing a Hybrid Cloud Storage Engine for HTAP
VLDB 2024 | Tobias Schmidt, Dominik Durner, Viktor Leis, and Thomas Neumann | August 26, 2024
Abstract
Businesses are increasingly demanding real-time analytics on up-to-date data. However, current solutions fail to efficiently combine transactional and analytical processing in a single system. Instead, they rely on extract-transform-load pipelines to transfer transactional data to analytical systems, which introduces a significant delay in the time-to-insight. In this paper, we address this need by proposing a new storage engine design for the cloud, called Colibri, that enables hybrid transactional and analytical processing beyond main memory. Colibri features a hybrid column-row store optimized for both workloads, leveraging emerging hardware trends. It effectively separates hot and cold data to accommodate diverse access patterns and storage devices. Our extensive experiments showcase up to 10x performance improvements for processing hybrid workloads on solid-state drives and cloud object stores.
Simple, Efficient, and Robust Hash Tables for Join Processing
DaMoN 2024 | Altan Birler, Tobias Schmidt, Philipp Fent, and Thomas Neumann | June 10, 2024
Abstract
Hash joins play a critical role in relational data processing and their performance is crucial for the overall performance of a database system. Due to the hard to predict nature of intermediate results, an ideal hash join implementation has to be both fast for typical queries and robust against unusual data distributions. In this paper, we present our simple, yet effective unchained in-memory hash table design. Unchained tables combine the techniques of build side partitioning, adjacency array layout, pipelined probes, Bloom filters, and software write-combine buffers to achieve significant improvements in n:m joins with skew, while preserving top-notch performance in 1:n joins. Our hash table outperforms open addressing by 2x on average in relational queries and both chaining and open addressing by up to 20x in graph processing queries.
2023
Exploiting Cloud Object Storage for High-Performance Analytics
VLDB 2023 | Dominik Durner, Viktor Leis, and Thomas Neumann | August 31, 2023
Abstract
Elasticity of compute and storage is crucial for analytical cloud database systems. All cloud vendors provide disaggregated object stores, which can be used as storage backend for analytical query engines. Until recently, local storage was unavoidable to process large tables efficiently due to the bandwidth limitations of the network infrastructure in public clouds. However, the gap between remote network and local NVMe bandwidth is closing, making cloud storage more attractive. This paper presents a blueprint for performing efficient analytics directly on cloud object stores. We derive cost- and performance-optimal retrieval configurations for cloud object stores with the first in-depth study of this foundational service in the context of analytical query processing. For achieving high retrieval performance, we present AnyBlob, a novel download manager for query engines that optimizes throughput while minimizing CPU usage. We discuss the integration of high-performance data retrieval in query engines and demonstrate it by incorporating AnyBlob in our database system Umbra. Our experiments show that even without caching, Umbra with integrated AnyBlob achieves similar performance to state-of-the-art cloud data warehouses that cache data on local SSDs while improving resource elasticity.
Bringing Compiling Databases to RISC Architectures
VLDB 2023 | Ferdinand Gruber, Maximilian Bandle, Alexis Engelke, Thomas Neumann, and Jana Giceva | August 30, 2023
Abstract
Current hardware development greatly influences the design deci- sions of modern database systems. For many modern performance-focused database systems, query compilation emerged as an integral part and different approaches for code generation evolved, making use of standard compilers, general-purpose compiler libraries, or domain-specific code generators. However, development primarily focused on the dominating x86-64 server architecture; but neglected current hardware developments towards other CPU architectures like ARM and other RISC architectures. Therefore, we explore the design space of code generation in database systems considering a variety of state-of-the-art compilation approaches with a set of qualitative and quantitative metrics. Based on our findings, we have developed a new code generator called FireARM for AArch64-based systems in our database system, Umbra. We identify general as well as architecture-specific challenges for custom code generation in databases and provide potential solutions to abstract or handle them. Furthermore, we present an extensive evaluation of different compilation approaches in Umbra on a wide variety of x86-64 and ARM machines. In particular, we compare quantitative performance characteristics such as compilation latency and query throughput. Our results show that using standard languages and compiler infrastructures reduces the barrier to employing query compilation and allows for high performance on big data sets, while domain-specific code generators can achieve a significantly lower compilation overhead and allow for better targeting of new architectures.
Asymptotically Better Query Optimization Using Indexed Algebra
VLDB 2023 | Philipp Fent, Guido Moerkotte, and Thomas Neumann | August 30, 2023
Abstract
Query optimization is essential for the efficient execution of queries. The necessary analysis, if we can and should apply optimizations and transform the query plan, is already challenging. Traditional techniques focus on the availability of columns at individual operators, which does not scale for analysis of data flow through the query. Tracking available columns per operator takes quadratic space, which can result in multi-second optimization time for deep algebra trees. Instead, we need to re-think the naive algebra representation to efficiently support data flow analysis. In this paper, we introduce Indexed Algebra, a novel representation of relational algebra that makes common optimization tasks efficient. Indexed Algebra enables efficient reasoning with an auxiliary index structure based on link/cut trees that support dynamic updates and queries in O(log n). This approach not only improves the asymptotic complexity, but also allows elegant and concise formulations for the data flow questions needed for query optimization. While large queries see theoretically unbounded improvements, Indexed Algebra also improves optimization time of the relatively harmless queries of TPC-H and TPC-DS by more than 1.8x.
Relation-Based In-Database Stream Processing
CDMS 2023 | Christian Winter, Alfons Kemper, and Thomas Neumann | August 28, 2023
Abstract
Data analytics pipelines are growing increasingly diverse, with relevant data being split across multiple systems and processing modes. In particular, the analysis of data streams, i.e., high-velocity ephemeral data, is attracting growing interest and hasled to the development of specialized stream processing engines. However, evaluating complex queries combining such ephemeral streams with historic data in a single system remains challenging. In this paper, we devise a novel stream processing technique that allows users to run ad hoc queries that combine streams and history tables in a relational database system. The backbone of our approach is a specialized ring-buffered relation, which allows for high ease of integration for existing database systems. We highlight the applicability of our approach by integrating it into the Umbra database system and demonstrate its performance against dedicated stream processing engines, outperforming them consistently for analytical workloads.
Exploiting Code Generation for Efficient LIKE Pattern Matching
ADMS 2023 | Adrian Riedl, Philipp Fent, Maximilian Bandle, and Thomas Neumann | August 28, 2023
Abstract
Efficiently evaluating text pattern matching is one of the most common computationally expensive tasks in data processing pipelines. Especially when dealing with text-heavy real-world data, evaluating even simple LIKE predicates is costly. Despite the abundance of text and the frequency of string-handling expressions in real-world queries, processing is an afterthought for most systems. We argue that we must instead properly integrate text processing into the flow of DBMS query execution. In this work, we propose a code generation approach that specifically tailors the generated code to the given pattern and matching algorithm and integrates cleanly into DBMS query compilation. In addition, we introduce a generalized SSE search algorithm that uses a sequence of SSE instructions to compare packed strings in the generated code to efficiently locate longer input patterns. Our approach of generating specialized code for each pattern eliminates the overhead of interpreting the pattern for each tuple. As a result, we improve the performance of LIKE pattern matching by up to 2.5×, demonstrating that code generation can significantly improve the efficiency of LIKE predicate evaluation in DBMSs.
Blue Elephants Inspecting Pandas: Inspection and Execution of Machine Learning Pipelines in SQL
EDBT 2023 | Maximilian E. Schüle, Luca Scalerandi, Alfons Kemper, and Thomas Neumann | March 28, 2023
Abstract
Data preprocessing, the step of transforming data into a suitable format for training a model, rarely happens within database systems but rather in external Python libraries and thus requires extraction from the database systems first. However, database systems are tuned for efficient data access and offer aggregate functions to calculate the distribution frequenciesnecessary to detect the under- or overrepresentation of a certain value within the data (bias). We argue that database systems with SQL are capable of executing machine learning pipelines as well as discovering technical biases---introduced by data preprocessing---efficiently. Therefore, we present a set of SQL queries to cover data preprocessing and data inspection: During preprocessing, we annotate the tuples with an identifier to compute the distribution frequency of columns. To inspect distribution changes, we join the preprocessed dataset with the original one on the tuple identifier and use aggregate functions to count the number of occurrences per sensitive column. This allows us to detect operations which filter out tuples and thus introduce a technical bias even for columns preprocessing has removed. To automatically generate such queries, our implementation extends the mlinspect project to transpile existing data preprocessing pipelines written in Python to SQL queries, while maintaining detailed inspection results using views orcommon table expressions (CTEs). The evaluation proves that a modern beyond main-memory database system, i.e. Umbra, accelerates the runtime for preprocessing and inspection. Even PostgreSQL as a disk-based database system shows similar performance for inspection to Umbra when materialising views.
Communication-Optimal Parallel Reservoir Sampling
BTW 2023 | Christian Winter, Moritz Sichert, Altan Birler, Thomas Neumann, and Alfons Kemper | March 6, 2023
Abstract
When evaluating complex analytical queries on high-velocity data streams, many systems cannot run those queries on all elements of a stream. Sampling is a widely used method to reduce the system load by replacing the input with a representative yet manageable subset. For unbounded data, reservoir sampling generates a fixed-size uniform sample independent of the input cardinality. However, the collection of reservoir samples itself can already be a bottleneck for high-velocity data.In this paper, we introduce a technique that allows fully parallelizing reservoir sampling for many-core architectures. Our approach relies on the efficient combination of thread-local samples taken over chunks of the input without necessitating communication during the sampling phase and with minimal communication when merging. We show how our efficient merge guarantees uniform random samples while allowing data to be distributed over worker threads arbitrarily. Our analysis of this approach within the Umbra database system demonstrates linear scaling along the available threads and the ability to sustain high-velocity workloads.
Seamless Integration of Parquet Files into Data Processing
BTW 2023 | Alice Rey, Michael Freitag, and Thomas Neumann | March 6, 2023
Abstract
Relational database systems are still the most powerful tool for data analysis. However, the steps necessary to bring existing data into the database make them unattractive for data exploration, especially when the data is stored in data lakes where users often use Parquet files, a binary column-oriented file format.This paper presents a fast Parquet framework that tackles these problems without costly ETL steps. We incrementally collect information during query execution.We create statistics that enhance future queries. In addition, we split the file into chunks for which we store the data ranges. We call these synopses. They allow us to skip entire sections in future queries.We show that these techniques only add minor overhead to the first query and are of benefit for future requests.Our evaluation demonstrates that our implementation can achieve comparable results to database relations and that we can outperform existing systems by up to an order of magnitude.
2022
Practical Planning and Execution of Groupjoin and Nested Aggregates
VLDBJ | Philipp Fent, Altan Birler, and Thomas Neumann | October 22, 2022
Abstract
Groupjoins combine execution of a join and a subsequent group-by. They are common in analytical queries and occur in about 1/8 of the queries in TPC-H and TPC-DS. While they were originally invented to improve performance, efficient parallel execution of groupjoins can be limited by contention in many-core systems. Efficient implementations of groupjoins are highly desirable, as groupjoins are not only used to fuse group-by and join, but are also useful to efficiently execute nested aggregates. For these, the query optimizer needs to reason over the result of aggregation to optimally schedule it. Traditional systems quickly reach their limits of selectivity and cardinality estimations over computed columns and often treat group-by as an optimization barrier. In this paper, we present techniques to efficiently estimate, plan, and execute groupjoins and nested aggregates. We propose four novel techniques, aggregate estimates to predict the result distributions of aggregates, parallel groupjoin execution for scalable execution of groupjoins, index groupjoins, and a greedy eager aggregation optimization technique that introduces nested preaggregations to significantly improve execution plans. The resulting system has improved estimates, better execution plans, and a contention-free evaluation of groupjoins, which speeds up TPC-H and TPC-DS queries significantly.
A Scalable and Generic Approach to Range Joins
VLDB 2022 | Maximilian Reif and Thomas Neumann | September 5, 2022
Abstract
Analytical database systems provide great insights into large datasets and are an excellent tool for data exploration and analysis. A central pillar of query processing is the efficient evaluation of equi-joins, typically with linear-time algorithms (e.g. hash joins). However, for many use-cases with location and temporal data, non-equi joins, like range joins, occur in queries. Without optimizations, this typically results in nested loop evaluation with quadratic complexity. This leads to unacceptable query execution times. Different mitigations have been proposed in the past, like partitioning or sorting the data. While these allow for handling certain classes of queries, they tend to be restricted in the kind of queries they can support. And, perhaps even more importantly, they do not play nice with additional equality predicates that typically occur within a query and that have to be considered, too. In this work, we present a kd-tree-based, multi-dimension range join that supports a very wide range of queries, and that can exploit additional equality constraints. This approach allows us to handle large classes of queries very efficiently, with negligible memory overhead, and it is suitable as a general-purpose solution for range queries in database systems. The join algorithm is fully parallel, both during the build and the probe phase, and scales to large problem instances and high core counts. We demonstrate the feasibility of this approach by integrating it into our database system Umbra and performing extensive experiments with both large real world data sets and with synthetic benchmarks used for sensitivity analysis. In our experiments, it outperforms hand-tuned Spark code and all other database systems that we have tested.
On-Demand State Separation for Cloud Data Warehousing
VLDB 2022 | Christian Winter, Jana Giceva, Thomas Neumann, and Alfons Kemper | September 5, 2022
Abstract
Moving data analysis and processing to the cloud is no longer reserved for a few companies with petabytes of data. Instead, the flexibility of on-demand resources is attracting an increasing number of customers with small to medium-sized workloads. These workloads do not occupy entire clusters but can run on single worker machines. However, picking the right worker for the job is challenging. Abstracting from worker machines, e.g., using stateless architectures, introduces overheads impacting performance. Solutions without stateless architectures resort to query restarts in the event of an adverse worker matching, wasting already achieved progress.
In this paper, we propose migrating queries between workers by introducing on-demand state separation. Using state separation only when required enables maximum flexibility and performance while keeping already achieved progress. To derive the requirements for state separation, we first analyze the query state of medium-sized workloads on the example of TPC-DS SF100. Using this, we analyze the cost and describe the constraints necessary for state separation on such a workload. Furthermore, we describe the design and implementation of on-demand state separation in a compiling database system. Finally, using this implementation, we show the feasibility of our approach on TPC-DS and give a detailed analysis of the cost of query migration and state separation.
Efficiently Compiling Dynamic Code for Adaptive Query Processing
ADMS 2022 | Tobias Schmidt, Philipp Fent, and Thomas Neumann | September 5, 2022
Abstract
Query optimization is one of the key challenges in modern database systems. With sophisticated data processing techniques like query compilation or vectorization, a good execution plan is essential for high performance. Yet, finding the optimal implementation for a query upfront is difficult as optimizers must rely on cardinality estimations and coarse cost models for the underlying hardware. Adaptive Query Processing overcomes these limitations: We evaluate different implementations for the same query during execution and choose the best-performing implementation based on accurate runtime measurements. However, compiling database systems cannot easily modify the generated code, and recompiling queries is prohibitively expensive. We propose a novel compilation technique — Dynamic Blocks — that avoids recompilations by embedding code fragments for all variants into the generated code. We integrate the approach into our research system Umbra and implement two dynamic optimizations for reordering joins and adapting selections during execution. Our results show that Adaptive Query Processing improves the runtime of data-centric code by more than 2×.
User-Defined Operators: Efficiently Integrating Custom Algorithms into Modern Databases
VLDB 2022 | Moritz Sichert and Thomas Neumann | September 5, 2022
Abstract
In recent years, complex data mining and machine learning algorithms have become more common in data analytics. Several specialized systems exist to evaluate these algorithms on ever-growing data sets, which are built to efficiently execute different types of complex analytics queries. However, using these various systems comes at a price. Moving data out of traditional database systems is often slow as it requires exporting and importing data, which is typically performed using the relatively inefficient CSV format. Additionally, database systems usually offer strong ACID guarantees, which are lost when adding new, external systems. This disadvantage can be detrimental to the consistency of the results. Most data scientists still prefer not to use classical database systems for data analytics. The main reason why RDBMS are not used is that SQL is difficult to work with due to its declarative and set-oriented nature, and is not easily extensible. We present User-Defined Operators (UDOs) as a concept to include custom algorithms into modern query engines. Users can write idiomatic code in the programming language of their choice, which is then directly integrated into existing database systems. We show that our implementation can compete with specialized tools and existing query engines while retaining all beneficial properties of the database system.
Memory-Optimized Multi-Version Concurrency Control for Disk-Based Database Systems
VLDB 2022 | Michael Freitag, Alfons Kemper, and Thomas Neumann | September 5, 2022
Abstract
Pure in-memory database systems offer outstanding performance but degrade heavily if the working set does not fit into DRAM, which is problematic in view of declining main memory growth rates. In contrast, recently proposed memory-optimized disk-based systems such as Umbra leverage large in-memory buffers for query processing but rely on fast solid-state disks for persistent storage. They offer near in-memory performance while the working set is cached, and scale gracefully to arbitrarily large data sets far beyond main memory capacity. Past research has shown that this architecture is indeed feasible for read-heavy analytical workloads.
We continue this line of work in the following paper, and present a novel multi-version concurrency control approach that enables a memory-optimized disk-based system to achieve excellent performance on transactional workloads as well. Our approach exploits that the vast majority of versioning information can be maintained entirely in-memory without ever being persisted to stable storage, which minimizes the overhead of concurrency control. Large write transactions for which this is not possible are extremely rare, and handled transparently by a lightweight fallback mechanism. Our experiments show that the proposed approach achieves transaction throughput up to an order of magnitude higher than competing disk-based systems, confirming its viability in a real-world setting.
Recursive SQL and GPU-support for in-database machine learning.
Distributed Parallel Databases | Maximilian E. Schüle, Harald Lang, Maximilian Springer, Alfons Kemper, Thomas Neumann, and Stephan Günnemann | July 9, 2022
Abstract
In machine learning, continuously retraining a model guarantees accurate predictions based on the latest data as training input. But to retrieve the latest data from a database, time-consuming extraction is necessary as database systems have rarely been used for operations such as matrix algebra and gradient descent. In this work, we demonstrate that SQL with recursive tables makes it possible to express a complete machine learning pipeline out of data preprocessing, model training and its validation. To facilitate the specification of loss functions, we extend the code-generating database system Umbra by an operator for automatic differentiation for use within recursive tables: With the loss function expressed in SQL as a lambda function, Umbra generates machine code for each partial derivative. We further use automatic differentiation for a dedicated gradient descent operator, which generates LLVM code to train a user-specified model on GPUs. We fine-tune GPU kernels at hardware level to allow a higher throughput and propose non-blocking synchronisation of multiple units. In our evaluation, automatic differentiation accelerated the runtime by the number of cached subexpressions compared to compiling each derivative separately. Our GPU kernels with independent models allowed maximal throughput even for small batch sizes, making machine learning pipelines within SQL more competitive.
LLVM Code Optimisation for Automatic Differentiation
DEEM 2022 | Maximilian E. Schüle, Maximilian Springer, Alfons Kemper, and Thomas Neumann | June 12, 2022
Abstract
Both forward and reverse mode automatic differentiation derive a model function as used for gradient descent automatically. Reverse mode calculates all derivatives in one run, whereas forward mode requires rerunning the algorithm with respect to every variable for which the derivative is needed. To allow for in-database machine learning, we have integrated automatic differentiation as an SQL operator inside the Umbra database system. To benchmark code-generation to GPU, we implement forward as well as reverse mode automatic differentiation. The inspection of the optimised LLVM code shows that nearly the same machine code is executed after the generated LLVM code has been optimised. Thus, both modes yield similar runtimes but different compilation times.
ArrayQL Integration into Code-Generating Database Systems
EDBT 2022 | Maximilian E. Schüle, Tobias Götz, Alfons Kemper, and Thomas Neumann | March 29, 2022
Abstract
Array database systems offer a declarative language for array-based access on multidimensional data. Although ArrayQL formulates the operators for a standardised query language, the corresponding syntax is not fully defined nor integrated in a productive system. Furthermore, we see potential in a uniform array query language to fill the gap between linear and relational algebra. This study explains the integration of ArrayQL inside a relational database system, either addressable through a separate query interface or integrated into SQL as user-defined functions. With a relational database system as the target, we inherit the benefits such as query optimisation and multi-version concurrency control by design. Apart from SQL, having another query language allows processing the data without extraction or transformation out of its relational form. This is possible as we work on a relational array representation, for which we translate each ArrayQL operator into relational algebra. This study provides an extended ArrayQL grammar specification to address each ArrayQL operator. In our evaluation, ArrayQL within Umbra computes matrix operations faster than state of the art database extensions and outperforms traditional array database systems on predicate evaluation and aggregations.
B²-Tree: Page-Based String Indexing in Concurrent Environments
Datenbank-Spektrum | Josef Schmeißer, Maximilian E. Schüle, Thomas Neumann, and Alfons Kemper | February 21, 2022
Abstract
Recently proposed index structures, that combine trie-based and comparison-based search mechanisms, considerably improve retrieval throughput for in-memory database systems. However, most of these index structures allocate small memory chunks when required. This stands in contrast to block-based index structures, that are necessary for disk-accesses of beyond main-memory database systems such as Umbra. We therefore present the B2-tree. The outer structure is identical to that of an ordinary B+-tree. It still stores elements in a dense array in sorted order, enabling efficient range scan operations. However, B2-tree is composed of multiple trees, each page integrates another trie-based search tree, which is used to determine a small memory region where a sought entry may be found. An embedded tree thereby consists of decision nodes, which operate on a single byte at a time, and span nodes, which are used to store common prefixes. This architecture usually accesses fewer cache lines than a vanilla B+-tree as shown in our performance evaluation. As a result, the B2-tree answers point queries considerably faster. Concurrent access to B2-tree pages are managed by an optimistic locking protocol which results in high utilization of the available hardware resources. Our evaluation of read-write workloads attests more than competitive performance for the B2-tree compared to a traditional B+-tree.
2021
B²-Tree: Cache-Friendly String Indexing within B-Trees
BTW 2021 | Josef Schmeißer, Maximilian E. Schüle, Thomas Neumann, and Alfons Kemper | September 13, 2021
Abstract
Recently proposed index structures, that combine trie-based and comparison-based search mechanisms, considerably improve retrieval throughput for in-memory database systems. However, most of these index structures allocate small memory chunks when required. This stands in contrast to block-based index structures, that are necessary for disk-accesses of beyond main-memory database systems such as Umbra. We therefore present the B²-tree. The outer structure is identical to that of an ordinary B+-tree. It still stores elements in a dense array in sorted order, enabling efficient range scan operations. However, B²-tree is composed of multiple trees, each page integrates another trie-based search tree, which is used to determine a small memory region where a sought entry may be found. An embedded tree thereby consists of decision nodes, which operate on a single byte at a time, and span nodes, which are used to store common prefixes. This architecture usually accesses fewer cache lines than a vanilla B+-tree as our performance evaluation proved. As a result, the B²-tree is able to answer point queries considerably faster.
Umbra as a Time Machine
BTW 2021 | Lukas Karnowski, Maximilian E. Schüle, Alfons Kemper, and Thomas Neumann | September 13, 2021
Abstract
Online encyclopaedias such as Wikipedia rely on incremental edits that change text strings marginally. To support text versioning inside of the Umbra database system, this study presents the implementation of a dedicated data type. This versioning data type is designed for maximal throughput as it stores the latest string as a whole and computes previous ones using backward diffs. Using this data type for Wikipedia articles, we achieve a compression rate of up to 11.9 % and outperform the traditional text data type, when storing each version as one tuple individually, by an order of magnitude.
A Practical Approach to Groupjoin and Nested Aggregates
VLDB 2021 | Philipp Fent and Thomas Neumann | August 19, 2021
Abstract
Groupjoins, the combined execution of a join and a subsequent group by, are common in analytical queries, and occur in about 1/8 of the queries in TPC-H and TPC-DS. While they were originally invented to improve performance, efficient parallel execution of groupjoins can be limited by contention, which limits their usefulness in a many-core system. Having an efficient implementation of groupjoins is highly desirable, as groupjoins are not only used to fuse group by and join but are also introduced by the unnesting component of the query optimizer to avoid nested-loops evaluation of aggregates. Furthermore, the query optimizer needs be able to reason over the result of aggregation in order to schedule it correctly. Traditional selectivity and cardinality estimations quickly reach their limits when faced with computed columns from nested aggregates, which leads to poor cost estimations and thus, suboptimal query plans. In this paper, we present techniques to efficiently estimate, plan, and execute groupjoins and nested aggregates. We propose two novel techniques, aggregate estimates to predict the result distribution of aggregates, and parallel groupjoin execution for a scalable execution of groupjoins. The resulting system has significantly better estimates and a contention-free evaluation of groupjoins, which can speed up some TPC-H queries up to a factor of 2.
In-Database Machine Learning with SQL on GPUs
SSDBM 2021 | Maximilian E. Schüle, Harald Lang, Maximilian Springer, Alfons Kemper, Thomas Neumann, and Stephan Günnemann | July 6, 2021
Abstract
In machine learning, continuously retraining a model guarantees accurate predictions based on the latest data as training input. But to retrieve the latest data from a database, time-consuming extraction is necessary as database systems have rarely been used for operations such as matrix algebra and gradient descent. In this work, we demonstrate that SQL with recursive tables makes it possible to express a complete machine learning pipeline out of data preprocessing, model training and its validation. To facilitate the specification of loss functions, we extend the code-generating database system Umbra by an operator for automatic differentiation for use within recursive tables: With the loss function expressed in SQL as a lambda function, Umbra generates machine code for each partial derivative. We further use automatic differentiation for a dedicated gradient descent operator, which generates LLVM code to train a user-specified model on GPUs. We fine-tune GPU kernels at hardware level to allow a higher throughput and propose non-blocking synchronisation of multiple units. In our evaluation, automatic differentiation accelerated the runtime by the number of cached subexpressions compared to compiling each derivative separately. Our GPU kernels with independent models allowed maximal throughput even for small batch sizes, making machine learning pipelines within SQL more competitive.
ArrayQL for Linear Algebra within Umbra
SSDBM 2021 | Maximilian E. Schüle, Tobias Götz, Alfons Kemper, and Thomas Neumann | July 6, 2021
Abstract
Array database systems offer a declarative language for array-based access on multidimensional data. This study explains the integration of ArrayQL inside a relational database system, either addressable through a separate query interface or integrated into SQL as user-defined functions. With a relational database system as the target, we inherit the benefits such as query optimisation and multi-version concurrency control by design. Apart from SQL, having another query language allows processing the data without extraction or transformation out of its relational form. This is possible as we work on a relational array representation, for which we translate each ArrayQL operator into relational algebra. In our evaluation, ArrayQL within Umbra computes matrix operations faster than state of the art database extensions.
JSON Tiles: Fast Analytics on Semi-Structured Data
SIGMOD 2021 | Dominik Durner, Viktor Leis, and Thomas Neumann | June 25, 2021
Abstract
Developers often prefer flexibility over upfront schema design, making semi-structured data formats such as JSON increasingly popular. Large amounts of JSON data are therefore stored and analyzed by relational database systems. In existing systems, however, JSON's lack of a fixed schema results in slow analytics. In this paper, we present JSON tiles, which, without losing the flexibility of JSON, enables relational systems to perform analytics on JSON data at native speed. JSON tiles automatically detects the most important keys and extracts them transparently - often achieving scan performance similar to columnar storage. At the same time, JSON tiles is capable of handling heterogeneous and changing data. Furthermore, we automatically collect statistics that enable the query optimizer to find good execution plans. Our experimental evaluation compares against state-of-the-art systems and research proposals and shows that our approach is both robust and efficient.
To Partition, or Not to Partition, That is the Join Question in a Real System
SIGMOD 2021 | Maximilian Bandle, Jana Giceva, and Thomas Neumann | June 25, 2021
Abstract
An efficient implementation of a hash join has been a highly researched problem for decades. Recently, the radix join has been shown to have superior performance over the alternatives (e.g., the non-partitioned hash join), albeit on synthetic microbenchmarks. Therefore, it is unclear whether one can simply replace the hash join in an RDBMS or use the radix join as a performance booster for selected queries. If the latter, it is still unknown when one should rely on the radix join to improve performance. In this paper, we address these questions, show how to integrate the radix join in Umbra, a code-generating DBMS, and make it competitive for selective queries by introducing a Bloom-filter based semi-join reducer. We have evaluated how well it runs when used in queries from more representative workloads like TPC-H. Surprisingly, the radix join brings a noticeable improvement in only one out of all 59 joins in TPC-H. Thus, with an extensive range of microbenchmarks, we have isolated the effects of the most important workload factors and synthesized the range of values where partitioning the data for the radix join pays off. Our analysis shows that the benefit of data partitioning quickly diminishes as soon as we deviate from the optimal parameters, and even late materialization rarely helps in real workloads. We thus, conclude that integrating the radix join within a code-generating database rarely justifies the increase in code and optimizer complexity and advise against it for processing real-world workloads.
Building Advanced SQL Analytics From Low-Level Plan Operators
SIGMOD 2021 | André Kohn, Viktor Leis, and Thomas Neumann | June 25, 2021
Abstract
Analytical queries virtually always involve aggregation and statistics. SQL offers a wide range of functionalities to summarize data such as associative aggregates, distinct aggregates, ordered-set aggregates, grouping sets, and window functions. In this work, we propose a unified framework for advanced statistics that composes all flavors of complex SQL aggregates from low-level plan operators. These operators can reuse materialized intermediate results, which decouples monolithic aggregation logic and speeds up complex multi-expression queries. The contribution is therefore twofold: our framework modularizes aggregate implementations, and outperforms traditional systems whenever multiple aggregates are combined. We integrated our approach into the high-performance database system Umbra and experimentally show that we compute complex aggregates faster than the state-of-the-art HyPer system.
Self-Tuning Query Scheduling for Analytical Workloads
SIGMOD 2021 | Benjamin Wagner, André Kohn, and Thomas Neumann | June 25, 2021
Abstract
Most database systems delegate scheduling decisions to the operating system. While such an approach simplifies the overall database design, it also entails problems. Adaptive resource allocation becomes hard in the face of concurrent queries. Furthermore, incorporating domain knowledge to improve query scheduling is difficult. To mitigate these problems, many modern systems employ forms of task-based parallelism. The execution of a single query is broken up into small, independent chunks of work (tasks). Now, fine-grained scheduling decisions based on these tasks are the responsibility of the database system. Despite being commonplace, little work has focused on the opportunities arising from this execution model. In this paper, we show how task-based scheduling in database systems opens up new areas for optimization. We present a novel lock-free, self-tuning stride scheduler that optimizes query latencies for analytical workloads. By adaptively managing query priorities and task granularity, we provide high scheduling elasticity. By incorporating domain knowledge into the scheduling decisions, our system is able to cope with workloads that other systems struggle with. Even at high load, we retain near optimal latencies for short running queries. Compared to traditional database systems, our design often improves tail latencies by more than 10x.
Tidy Tuples and Flying Start: Fast Compilation and Fast Execution of Relational Queries in Umbra
VLDBJ | Timo Kersten, Viktor Leis, and Thomas Neumann | June 2, 2021
Abstract
Although compiling queries to efficient machine code has become a common approach for query execution, a number of newly-created database system projects still refrain from using compilation. It is sometimes claimed that the intricacies of code generation make compilation-based engines too complex. Also, a major barrier for adoption, especially for interactive ad-hoc queries, is long compilation time. In this paper, we examine all stages of compiling query execution engines and show how to reduce compilation overhead. We incorporate the lessons learned from a decade of generating code in HyPer into a design that manages complexity and yields high speed. First, we introduce a code generation framework that establishes abstractions to manage complexity, yet generates code in a single fast pass. Second, we present a program representation whose data structures are tuned to support fast code generation and compilation. Third, we introduce a new compiler backend that is optimized for minimal compile time, and simultaneously, yields superior execution performance to competing approaches, e.g., Volcano-style or bytecode interpretation. We implemented these optimizations in our database system Umbra to show that it is possible to unite fast compilation and fast execution. Indeed, Umbra achieves unprecedentedly low query latencies. On small data sets, it is even faster than interpreter engines like DuckDB and PostgreSQL. At the same time, on large data sets, its throughput is on par with the state-of-the-art compiling system HyPer.
Profiling Dataflow Systems on Multiple Abstraction Levels
ACM EuroSys 2021 | Alexander Beischl, Timo Kersten, Maximilian Bandle, Jana Giceva, and Thomas Neumann | April 27, 2021
Abstract
Dataflow graphs are a popular abstraction for describing computation, used in many systems for high-level optimization. For execution, dataflow graphs are lowered and optimized through layers of program representations down to machine instructions. Unfortunately, performance profiling such systems is cumbersome, as today's profilers present results merely at instruction and function granularity. This obfuscates the connection between profiles and high-level constructs, such as operators and pipelines, making interpretation of profiles an exercise in puzzling and deduction.
In this paper, we show how to profile compiling dataflow systems at higher abstraction levels. Our approach tracks the code generation process and aggregates profiling data to any abstraction level. This bridges the semantic gap to match the engineer's current information need and even creates a comprehensible way to report timing information within profiling data. We have evaluated this approach within our compiling DBMS Umbra, showing that the approach is generally applicable for compiling dataflow systems and can be implemented with high accuracy and reasonable overhead.
2020
Mosaic: A Budget-Conscious Storage Engine for Relational Database Systems
VLDB 2020 | Lukas Vogel, Alexander van Renen, Satoshi Imamura, Viktor Leis, Thomas Neumann, and Alfons Kemper | August 30, 2020
Abstract
Relational database systems are purpose-built for a specific storage device class (e.g., HDD, SSD, or DRAM). They do not cope well with the multitude of storage devices that are competitive at their price 'sweet spots'. To make use of different storage device classes, users have to resort to workarounds, such as storing data in different tablespaces. A lot of research has been done on heterogeneous storage frameworks for distributed big data query engines. These engines scale well for big data sets but are often CPU- or network-bound. Both approaches only maximize performance for previously purchased storage devices. We present Mosaic, a storage engine for scan-heavy workloads on RDBMS that manages devices in a tierless pool and provides device purchase recommendations for a specified workload and budget. In contrast to existing systems, Mosaic generates a performance/budget curve that is Pareto-optimal, along which the user can choose. Our approach uses device models and linear optimization to find a data placement solution that maximizes I/O throughput for the workload. Our evaluation shows that Mosaic provides a higher throughput at the same budget or a similar throughput at a lower budget than the state-of-the-art approaches of big data query engines and RDBMS.
Meet Me Halfway: Split Maintenance of Continuous Views
VLDB 2020 | Christian Winter, Tobias Schmidt, Thomas Neumann, and Alfons Kemper | August 30, 2020
Abstract
From Industry 4.0-driven factories to real-time trading algorithms, businesses depend on analytics on high-velocity real-time data. Often these analytics are performed not in dedicated stream processing engines but on views within a general-purpose database to combine current with historical data. However, traditional view maintenance algorithms are not designed with both the volume and velocity of data streams in mind.
In this paper, we propose a new type of view specialized for queries involving high-velocity inputs, called continuous view. The key component of continuous views is a novel maintenance strategy, splitting the work between inserts and queries. By performing initial parts of the view's query for each insert and the remainder at query time, we achieve both high input rates and low query latency. Further, we keep the memory overhead of our views small, independent of input velocity. To demonstrate the practicality of this strategy, we integrate continuous views into our Umbra database system. We show that split maintenance can outperform even dedicated stream processing engines on analytical workloads, all while still offering similar insert rates. Compared to modern materialized view maintenance approaches, such as deferred and incremental view maintenance, that often need to materialize expensive deltas, we achieve up to an order of magnitude higher insert throughput.
Adopting Worst-Case Optimal Joins in Relational Database Systems
VLDB 2020 | Michael Freitag, Maximilian Bandle, Tobias Schmidt, Alfons Kemper, and Thomas Neumann | August 30, 2020
Abstract
Worst-case optimal join algorithms are attractive from a theoretical point of view, as they offer asymptotically better runtime than binary joins on certain types of queries. In particular, they avoid enumerating large intermediate results by processing multiple input relations in a single multiway join. However, existing implementations incur a sizable overhead in practice, primarily since they rely on suitable ordered index structures on their input. Systems that support worst-case optimal joins often focus on a specific problem domain, such as read-only graph analytic queries, where extensive precomputation allows them to mask these costs.
In this paper, we present a comprehensive implementation approach for worst-case optimal joins that is practical within general-purpose relational database management systems supporting both hybrid transactional and analytical workloads. The key component of our approach is a novel hash-based worst-case optimal join algorithm that relies only on data structures that can be built efficiently during query execution. Furthermore, we implement a hybrid query optimizer that intelligently and transparently combines both binary and multi-way joins within the same query plan. We demonstrate that our approach far outperforms existing systems when worst-case optimal joins are beneficial while sacrificing no performance when they are not.
On another level: how to debug compiling query engines
DBTest 2020 | Timo Kersten and Thomas Neumann | June 19, 2020
Abstract
Compilation-based query engines generate and compile code at runtime, which is then run to get the query result. In this process there are two levels of source code involved: The code of the code generator itself and the code that is generated at runtime. This can make debugging quite indirect, as a fault in the generated code was caused by an error in the generator. To find the error, we have to look at both, the generated code and the code that generated it.
Current debugging technology is not equipped to handle this situation. For example, GNU’s gdb only offers facilities to inspect one source line, but not multiple source levels. Also, current debuggers are not able to reconstruct additional program state for further source levels, thus, context is missing during debugging.
In this paper, we show how to build a multi-level debugger for generated queries that solves these issues. We propose to use a time-travelling debugger to provide context information for compile-time and runtime, thus providing full interactive debugging capabilities for every source level. We also present how to build such a debugger with low engineering effort by combining existing tool chains.
Concurrent online sampling for all, for free
DaMoN 2020 | Altan Birler, Bernhard Radke, and Thomas Neumann | June 15, 2020
Abstract
Database systems rely upon statistical synopses for cardinality estimation. A very versatile and powerful method for estimation purposes is to maintain a random sample of the data. However, drawing a random sample of an existing data set is quite expensive due to the resulting random access pattern, and the sample will get stale over time. It is much more attractive to use online sampling, such that a fresh sample is available at all times, without additional data accesses. While clearly superior from a theoretical perspective, it was not clear how to efficiently integrate online sampling into a database system with high concurrent update and query load.
We introduce a novel highly scalable online sampling strategy that allows for sample maintenance with minimal overhead. We can trade off strict freshness guarantees for a significant boost in performance in many-core shared memory scenarios, which is ideal for estimation purposes. We show that by replacing the traditional periodical sample reconstruction in a database system with our online sampling strategy, we get virtually zero overhead in insert performance and completely eliminate the slow random I/O needed for sample construction.
Scalable and Robust Latches for Database Systems
DaMoN 2020 | Jan Böttcher, Viktor Leis, Jana Giceva, Thomas Neumann, and Alfons Kemper | June 15, 2020
Abstract
Multi-core scalability is one of the most important features for database systems running on today’s hardware. Not surprisingly, the implementation of locks is paramount to achieving efficient and scalable synchronization. In this work, we identify the key database-specific requirements for lock implementations and evaluate them using both micro-benchmarks and full-fledged database workloads. The results indicate that optimistic locking has superior performance in most workloads due to its minimal overhead and latency. By complementing optimistic locking with a pessimistic shared mode lock we demonstrate that we can also process HTAP workloads efficiently. Finally, we show how lock contention can be handled gracefully without slowing down the uncontended fast path or increasing space requirements by using a lightweight parking lot infrastructure.
2019
Experimental Study of Memory Allocation for High-Performance Query Processing
ADMS 2019 | Dominik Durner, Viktor Leis, and Thomas Neumann | August 26, 2019
Abstract
Somewhat surprisingly, the behavior of analytical query engines is crucially affected by the dynamic memory allocator used. Memory allocators highly influence performance, scalability, memory efficiency and memory fairness to other processes. In this work, we provide the first comprehensive experimental study that analyzes and explains the impact of memory allocation for high-performance query engines. We test five state-of-the-art dynamic memory allocators and discuss their strengths and weaknesses within our DBMS. The right allocator can increase the performance of TPC-DS (SF 100) by 2.7x on a 4-socket Intel Xeon server.
On the Impact of Memory Allocation on High-Performance Query Processing
DaMoN 2019 | Dominik Durner, Viktor Leis, and Thomas Neumann | July 1, 2019
Abstract
[Short Paper - Long Version at ADMS 2019] Somewhat surprisingly, the behavior of analytical query engines is crucially affected by the dynamic memory allocator used. Memory allocators highly influence performance, scalability, memory efficiency and memory fairness to other processes. In this work, we provide the first comprehensive experimental analysis on the impact of memory allocation for high-performance query engines. We test five state-of-the-art dynamic memory allocators and discuss their strengths and weaknesses within our DBMS. The right allocator can increase the performance of TPC-DS (SF 100) by 2.7xon a 4-socket Intel Xeon server.
Scalable Reservoir Sampling on Many-Core CPUs
ACM SIGMOD 2019 | Altan Birler | June 30, 2019
Abstract
Database systems need to be able to convert queries to efficient execution plans. As recent research has shown, correctly estimating cardinalities of subqueries is an important factor in the efficiency of the resulting plans. Many algorithms have been proposed in literature that utilize a random sample to estimate cardinalities. Thus, some modern database systems choose to store a materialized uniformly random sample for their relations. Such samples are built and refreshed when statistics are gathered, by loading uniformly random tuples from the relation in disk using random IO.
No False Negatives: Accepting All Useful Schedules in a Fast Serializable Many-Core System
ICDE 2019 | Dominik Durner and Thomas Neumann | April 9, 2019
Abstract
Concurrency control is one of the most performance critical steps in modern many-core database systems. Achieving higher throughput on multi-socket servers is difficult and many concurrency control algorithms reduce the amount of accepted schedules in favor of transaction throughput or relax the isolation level which introduces unwanted anomalies. Both approaches lead to unexpected transaction behavior that is hard to under-stand by the database users. We introduce a novel multi-version concurrency protocol that achieves high performance while reducing the number of aborted schedules to a minimum and providing the best isolation level. Our approach leverages the idea of a graph-based scheduler that uses the concept of conflict graphs. As conflict serializable histories can be represented by acyclic conflict graphs, our scheduler maintains the conflict graph and allows all transactions that keep the graph acyclic. All conflict serializable schedules can be accepted by such a graph-based algorithm due to the conflict graph theorem. Hence, only transaction schedules that really violate the serializability constraints need to abort. Our developed approach is able to accept the entire useful intersection of order preserving conflict serializable (OCSR) and recoverable (RC) schedules which are the two most desirable classes in terms of correctness and user experience. We show experimentally that our graph-based scheduler has very competitive throughput in pure transactional workloads while providing fewer aborts and improved user experience. Our multi-version extension helps to efficiently perform long-running read transactions on the same up-to-date database. Moreover, our graph-based scheduler can outperform the competitors on mixed workloads.
Making Compiling Query Engines Practical
TKDE | André Kohn, Viktor Leis, and Thomas Neumann | March 15, 2019
Abstract
Compiling queries to machine code is a very efficient way for executing queries. One often overlooked problem with compilation is the time it takes to generate machine code. Even with fast compilation frameworks like LLVM, generating machine code for complex queries often takes hundreds of milliseconds. Such durations can be a major disadvantage for workloads that execute many complex, but quick queries. To solve this problem, we propose an adaptive execution framework, which dynamically switches from interpretation to compilation. We also propose a fast bytecode interpreter for LLVM, which can execute queries without costly translation to machine code and dramatically reduces the query latency. Adaptive execution is fine-grained, and can execute code paths of the same query using different execution modes. Our evaluation shows that this approach achieves optimal performance in a wide variety of settings---low latency for small data sets and maximum throughput for large data sizes. Besides compilation time, we also focus on debugging, which is another important challenge of compilation-based query engines. To address this problem, we present a novel, database-specific debugger for compiling query engines.
Every Row Counts: Combining Sketches and Sampling for Accurate Group-By Result Estimates
CIDR 2019 | Michael Freitag and Thomas Neumann | January 13, 2019
Abstract
Database systems heavily rely upon cardinality estimates for finding efficient execution plans, and estimation errors can easily affect query execution times by large factors. One particularly difficult problem is estimating the result size of a group-by operator, or, in general, the number of distinct combinations of a set of attributes. In contrast to, e. g., estimating the selectivity of simple filter predicates, the resulting number of groups cannot be predicted reliably without examining the complete input. As a consequence, most existing systems have poor estimates for the number of distinct groups. However, scanning entire relations at optimization time is not feasible in practice. Also, precise group counts cannot be precomputed for every possible combination of attributes. For practical purposes, a cheap mechanism is thus required which can handle arbitrary attribute combinations efficiently and with high accuracy. In this work, we present a novel estimation framework that combines sketched full information over individual columns with random sampling to correct for correlation bias between attributes. This combination can estimate group counts for individual columns nearly perfectly, and for arbitrary column combinations with high accuracy. Extensive experiments show that these excellent results hold for both synthetic and real-world data sets. We demonstrate how this mechanism can be integrated into existing systems with low overhead, and how estimation time can be kept negligible by means of an efficient algorithm for sample scans.
2018
Everything You Always Wanted To Known About Compiled and Vectorized Queries But Were Afraid To Ask
VLDB 2018 | Timo Kersten, Viktor Leis, Alfons Kemper, Thomas Neumann, Andrew Pavlo, and Peter Boncz | September 13, 2018
Abstract
The query engines of most modern database systems are either based on vectorization or data-centric code generation. These two state-of-the-art query processing paradigms are fundamentally dif- ferent in terms of system structure and query execution code. Both paradigms were used to build fast systems. However, until today it is not clear which paradigm yields faster query execution, as many implementation-specific choices obstruct a direct comparison of ar- chitectures. In this paper, we experimentally compare the two mod- els by implementing both within the same test system. This allows us to use for both models the same query processing algorithms, the same data structures, and the same parallelization framework to ul- timately create an apples-to-apples comparison. We find that both are efficient, but have different strengths and weaknesses. Vector- ization is better at hiding cache miss latency, whereas data-centric compilation requires fewer CPU instructions, which benefits cache- resident workloads. Besides raw, single-threaded performance, we also investigate SIMD as well as multi-core parallelization and dif- ferent hardware architectures. Finally, we analyze qualitative dif- ferences as a guide for system architects.
Adaptive Optimization of Very Large Join Queries
ACM SIGMOD 2018 | Thomas Neumann and Bernhard Radke | June 10, 2018
Abstract
The use of business intelligence tools and other means to generate queries has led to great variety in the size of join queries. While most queries are reasonably small, join queries with up to a hundred relations are not that exotic anymore, and the distribution of query sizes has an incredible long tail. The largest real-world query that we are aware of accesses more than 4,000 relations. This large spread makes query optimization very challenging. Join ordering is known to be NP-hard, which means that we cannot hope to solve such large problems exactly. On the other hand most queries are much smaller, and there is no reason to sacrifice optimality there. This paper introduces an adaptive optimization framework that is able to solve most common join queries exactly, while simultaneously scaling to queries with thousands of joins. A key component there is a novel search space linearization technique that leads to near-optimal execution plans for large classes of queries. In addition, we describe implementation techniques that are necessary to scale join ordering algorithms to these extremely large queries. Extensive experiments with over 10 different approaches show that the new adaptive approach proposed here performs excellent over a huge spectrum of query sizes, and produces optimal or near-optimal solutions for most common queries.
LeanStore: In-Memory Data Management beyond Main Memory
ICDE 2018 | Viktor Leis, Michael Haubenschild, Alfons Kemper, and Thomas Neumann | April 17, 2018
Abstract
Disk-based database systems use buffer managers in order to transparently manage data sets larger than main memory. This traditional approach is effective at minimizing the number of I/O operations, but is also the major source of overhead in comparison with in-memory systems. To avoid this overhead, in-memory database systems therefore abandon buffer management altogether, which makes handling data sets larger than main memory very difficult. In this work, we revisit this fundamental dichotomy and design a novel storage manager that is optimized for modern hardware. Our evaluation, which is based on TPC-C and micro benchmarks, shows that our approach has little overhead in comparison with a pure in-memory system when all data resides in main memory. At the same time, like a traditional buffer manager, it is fully transparent and can manage very large data sets effectively. Furthermore, due to low-overhead synchronization, our implementation is also highly scalable on multi-core CPUs.
Adaptive Execution of Compiled Queries
ICDE 2018 | André Kohn, Viktor Leis, and Thomas Neumann | April 16, 2018
Abstract
Compiling queries to machine code is a very efficient way for executing queries. One often overlooked problem with compilation is the time it takes to generate machine code. Even with fast compilation frameworks like LLVM, generating machine code for complex queries often takes hundreds of milliseconds. Such durations can be a major disadvantage for workloads that execute many complex, but quick queries. To solve this problem, we propose an adaptive execution framework, which dynamically switches from interpretation to compilation. We also propose a fast bytecode interpreter for LLVM, which can execute queries without costly translation to machine code and dramatically reduces the query latency. Adaptive execution is fine-grained, and can execute code paths of the same query using different execution modes. Our evaluation shows that this approach achieves optimal performance in a wide variety of settings—low latency for small data sets and maximum throughput for large data sizes.
Contact
Let's Work Together. Get in Touch!
Address
Institut für Informatik
Lehrstuhl III: Datenbanksysteme (I3)
Boltzmannstraße 3
85748 Garching bei München
Germany
Research Opportunities
Contact us for Ph.D. positions, student theses, collaborations, and research opportunities.
Email UsStay Up To Date With Our Blog
A blog written by and for database architects with approx. quarterly articles. Read about the latest research in database systems, and be at the forefront in our field.
Go To Blog